A Systems-Based Approach to Digital Design & Operation
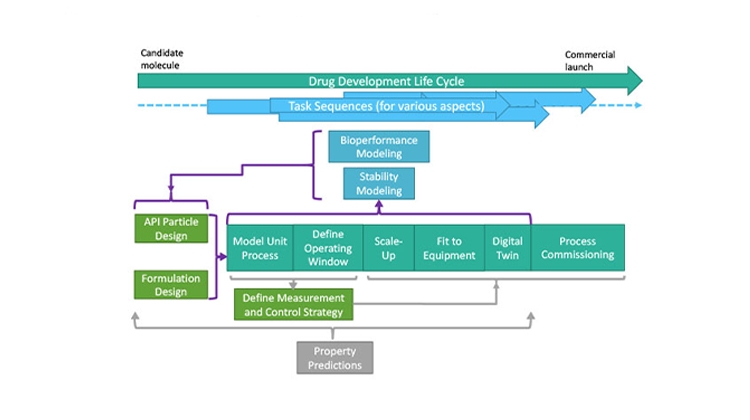
The Advanced Digital Design of Pharmaceutical Therapeutics (ADDoPT) project
Through a dedicated technical facilitation process, ADDoPT consortium members identified a need for, and developed, information flow for digital design and manufacture of pharmaceuticals, which can help the pharmaceutical industry adopt new technology and build the necessary innovation and leadership capacity to realize the potential of Industry 4.0 and similar initiatives around the world.
Industry 4.0 and Digitalization
The worldwide transformation of manufacturing through the increasing use of digitalization has been christened “the Fourth Industrial Revolution” or “Industry 4.0.”2, 3 Properly harnessed, digitalization has the potential to transform the future of manufacturing in all sectors, although progress varies widely across industry sectors and countries. The influential UK government–sponsored 2017 “Made Smarter Review”4 highlighted that UK industry in general is not taking advantage of a rapidly growing capability in industrial digitalization technology; therefore, the review’s authors recommended technology adoption and supporting the innovation and leadership necessary for the country to realize Industry 4.0’s potential.
Attitudes and digital readiness vary considerably across industrial sectors.5, 6. For example, in the oil and gas industry, process technologies and manufacturing processes are well developed,7 and modeling for optimization and control systems is an established practice to minimize production variations based on technology licensor, engineering, and operational constraints. In contrast, the operating context in pharmaceutical product manufacturing is very different. Absolute production volumes are relatively small, unit product costs and values are high (reflecting upfront development or acquisition cost, scale-up risks, and other factors), and products are typically produced in relatively short-run production campaigns with frequent product changeovers. Additionally, because the pharmaceutical industry has a large asset base for batch manufacturing that will persist for several decades, it’s important to maximize batch process value and quality and to build robustness into emerging flexible manufacturing solutions.
In the oil and gas industry, the impact of feedstocks on process and product quality is established and understood, and integrated digital approaches exist for plant design and operation. Conversely, because end-to-end pharmaceutical product manufacturing is complex, such links may not be readily established, and quality-critical links can differ between products. Unlike products in the oil and gas industry, each new pharmaceutical product is, in effect, a novel design and requires process optimization on production scale; also, although individual steps may be modeled, integrated digital approaches are not commonly available. With process-scale experimentation at an absolute premium, pharmaceutical manufacturers need to derive the necessary process insight, predictive models, and capacity to ensure quality in new ways.
In fact, pharmaceutical manufacturing technologies have not changed significantly in over 40 years. Though manufacturers are data-rich, they have yet to fully realize and pursue the potential of advanced process modeling and analytics to transform decision-making in process design and control. Process and product development are still predominantly based on a make-and-test approach, which requires long development cycles, nonrobust scale-ups, and inefficient processes. The industry has tolerated these limitations during the “blockbuster drug era,” but as this era ends,8 and as the need to realize the benefit of personalized medicines increases, new approaches are necessary. Furthermore, these approaches may open new possibilities such as holistically linking solid form, active pharmaceutical ingredient (API) physical properties, and drug product formulation and manufacture.9
As the pharmaceutical industry increasingly uses outsourcing/offshoring to reduce costs, it faces associated supply chain risks and mitigation costs. Personalized medicines require a shift from one-size-fits-all products and manufacturing processes toward greater flexibility in both. Regulators such as the US FDA and the European Medicines Agency (EMA) support quality by design (QbD),10 an approach that aims to ensure the quality of pharmaceutical products by employing statistical, analytical, and risk-management methodologies in the design, development, and manufacturing of these products. A goal of QbD is to ensure all sources of variability affecting a process are identified, explained, and managed through appropriate measures. This enables the finished product to consistently meet predefined characteristics from the start, ensuring it is right the first time. Current QbD approaches tend to be experimentally driven and therefore data-hungry and resource-intensive. Their uptake is further limited at present because it is generally not practical to extrapolate beyond experimental boundaries, and so there is limited opportunity to transfer knowledge and understanding gained to other scales.
To address these risks and costs, the pharmaceutical industry is exploring solutions such as flexible manufacturing and advanced process modeling and control; however, unlike other industries, such as oil and gas, the pharmaceutical industry does not currently routinely embed these approaches.
Advanced Digital Design
ADDoPT was established in response to challenges faced by the industry worldwide. The 12 collaboration partners included four multinational pharmaceutical companies (AstraZeneca, Bristol-Myers Squibb, GlaxoSmithKline [GSK], and Pfizer), three technology-based small- to medium-size enterprises (SMEs) (Process Systems Enterprise [PSE], Perceptive Engineering, and Britest), and five specialist academic and research organizations (Universities of Leeds, Cambridge, and Strathclyde; Cambridge Crystallographic Data Centre; and the Science and Technology Facilities Council’s Hartree Centre).
Consortium members worked across the pharmaceutical value chain to define a system for top-down, knowledge-driven digital design and control for drug products and their manufacturing processes. The aim was to integrate a wide range of predictive models and gain insight from industrial case studies at the four major pharmaceutical companies, allowing more targeted future experimentation, a better understanding of risk, and therefore better design and scale-up for robust products and processes.
Personalized medicines require a shift from one-size-fits-all products and manufacturing processes toward greater flexibility in both.
Digital design combines research insight and qualitative and quantitative mechanistic modeling to provide links between raw materials, manufacturing processes, and product performance to meet patients’ needs. It spans all unit operations, processes, and procedures during the manufacture of pharmaceutical products and their impacts, both upstream for product efficiency and process design, and downstream on product performance. ADDoPT’s objective was to “create virtual medicine manufacturing systems to make sure they are effective and efficient before creating them in the real world.”11
The consortium determined at the outset that to ensure a consistent and effective approach to digital design and manufacture of pharmaceutical products, an overarching system-based approach would be required to manage and combine information flows from the many facets of modeling and (where still necessary) experimentation. This information flow map becomes a significant enabler for guiding adoption of industrial digitalization technology, aligning with the Made Smarter Review recommendations and generally emerging trends in the global pharmaceutical sector.
Systems Framework–Based Innovation
Technology- and knowledge-driven partners worked within ADDoPT to advance the current state of process modeling and control for pharmaceutical processes, and to advantageously combine and integrate technologies based on a systems framework–based approach to understand and address the pharmaceutical industry’s needs. One benefit may be to stimulate systems thinking12 in pharmaceutical product and process development. By replacing the typically linear approach to development, the industry can potentially reduce risk; for example, a systems framework–based approach might prevent a repeat of the 1998 polymorphism issue that led to Ritonavir being temporarily withdrawn from the market.13
Current Best Practices and Areas for Development
Three ADDoPT industrial partners from the pharmaceutical industry (AstraZeneca, GSK, and Pfizer) were interviewed by Britest and PSE to establish to what extent digital modeling approaches are currently part of process development routes to introduce new therapeutic products (see Figure 1). The study’s scope comprised API particle manufacture and formulation of immediate-release oral dose products, and it explored links between manufacturing process development and bioperformance and stability. To keep the size of the study manageable, API synthesis was excluded.
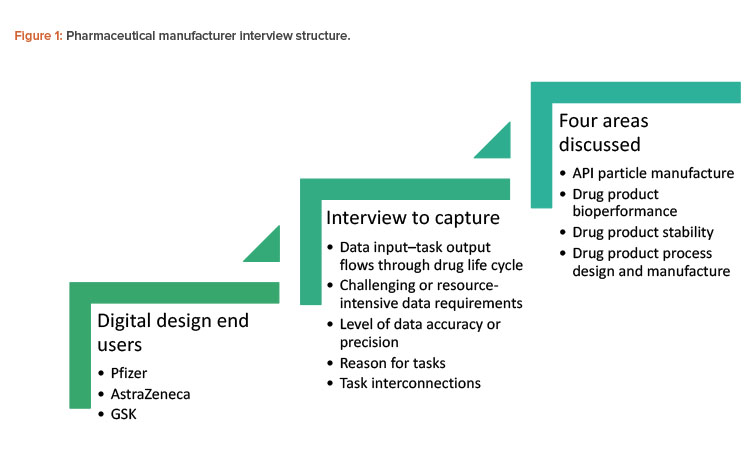
Generalized conclusions from the three companies interviewed can be summarized under three categories.
- Current practice: Workflows are primarily experimentally driven, progress is sequential (meaning, influenced by launch timeline), output is rarely used to revise earlier process steps to ultimately deliver a better result, and a large amount of confirmatory experimental work is undertaken to manage risk.
- Current influence of digital design techniques: These techniques—which are in development and not yet in mainstream use—are used to confirm, explain, or fit experimental data. They are not integrated into the overall product and process design approach.
- Opportunities provided by digital design techniques: First, task and decision-making flows are similar across the companies, which allows for identification of common tasks and/or decisions points. A standardized approach could streamline progress within organizations and make regulatory reviews more efficient. Second, data and information transfer points could be coordinated between business functional groups. Third, de-risking may potentially become more efficient than current practices. By using digital design techniques, manufacturers could build in an iterative approach to achieve a more robust overall process; identify risk sooner, which would enable the defining of mitigation strategies; and support better management of work and experimental timelines, as modeling could focus on promising and relevant operating windows.
To capture impressions from solution providers, the three SME businesses (Britest, PSE, and Perceptive Engineering) were also interviewed. The topics discussed with each company varied according to the nature of their solutions, and are outlined next.
For PSE and Britest, interviews addressed three main topics: (a) the business model for interacting with clients in the pharmaceutical industry; (b) the current status of tools that support ADDoPT-relevant key unit operations; and (c) the current and potential opportunities for the use of tools for bio-pharmaceutical/formulation design/drug product stability applications.
The interviews with a representative of Perceptive Engineering covered a series of questions:
- Where in the development life cycle do you normally engage with the client?
- Where in the life cycle would you like to engage?
- What is the normal pattern/program for a project?
- In the modeling process, what data do you need/get? What data would you like to get?
- How can you interface to a model process without actual plant data to build a control strategy?
- Can you provide feedback and add value to the clients’ development process?
From these interviews, common themes and conclusions emerged. Service providers usually become involved with clients only after key directional decisions are made, which limits the value that service providers can add for the client. Service providers are engaged with/by research and development, rather than the manufacturing communities (although some localized progress has occurred). Digital design approaches developed for the pharmaceutical sector have significant potential for applications in other high-value product sectors.
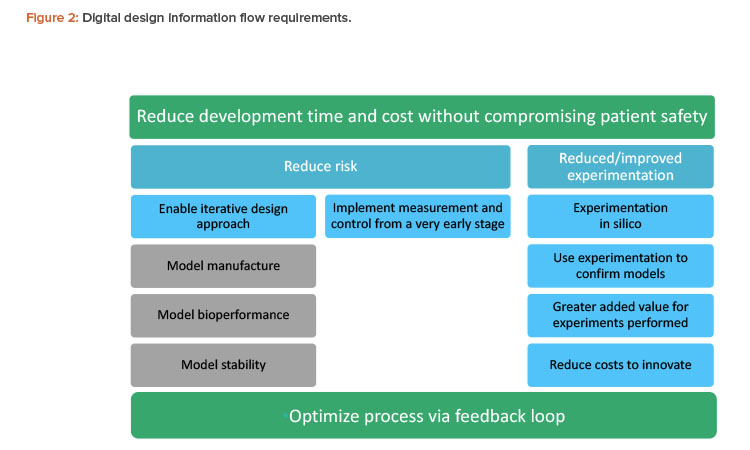
Information Flow Requirements
Commercially launching a new pharmaceutical product is a complex and lengthy process, which involves nearly all of the organization and typically extends into the supply chain, particularly if parts of production have been outsourced to contract manufacturing organizations.
The goal of ADDoPT is to significantly streamline and expedite this complex process while enhancing (or at least not compromising) patient safety. Therefore, any approach to digital design and manufacture must consider not only the end-to-end pharmaceutical manufacturing process but also the wider system, including product design and performance.
Clearly, many groups, and people from many functions and disciplines, must share information effectively. Therefore, those devising a digital approach should focus on information flow instead of a linear, project plan–based workflow. The difference lies in the promotion of systems thinking rather than ticking off a list of discrete deliverables.
Successfully harnessing new digitally enabled approaches is not just a matter of technical implementation; it involves a fundamental paradigm shift for the pharmaceutical industry, moving away from its traditional experimentally based approach to a new design-and-make framework. Just as new enabling modeling and control platforms are designed to be integrated across the pharmaceutical workflow, product and process design and control decisions must consider all relevant upstream and downstream impacts and dependencies, rather than merely seeking a local optimum. For instance, API crystallization might conventionally be optimized to deliver a given average particle size, but simply replicating this specified value from one batch to another does not necessarily ensure trouble-free downstream processing.
There is growing recognition of the need to characterize materials more richly, and to analyze the connection between molecular and material properties at one end through to process and product performance at the other. Developments in statistical and mechanistic modeling approaches, the ability to harness big data, and effective cross-industry collaboration over matters such as the emergent manufacturing classification system14 can all help put the pieces of the puzzle together.
Committing to a digital (in silico) approach offers many potential benefits. By using in silico experimentation, the pharmaceutical industry can speed up timelines and consider all or a larger subset of a system’s factors, while minimizing and better directing the set of practical experiments required to validate the digital model. An in silico approach to design of experiments also reduces risk by eliminating the practical cost and time constraints that currently lead us to limit experiments to the subset of variables considered most likely to prove critical; in digital experiments, we can routinely explore all potentially critical variables. In silico modeling makes a more broadly applicable QbD approach practical and resource-efficient while also minimizing the quantity of API required for experiments in which the API is difficult to produce or obtain.
New Ways of Thinking and Working
The pharmaceutical industry needs to integrate process development with considerations of patient safety, efficacy, and manufacturability. If this is done successfully, the result will be optimized, more consistent, and more robust products from the outset. A holistic approach helps manufacturers first determine the product performance attributes required and then design the process to deliver the required product. Figure 2 summarizes the overall requirements for a successful information flow for digital design and manufacture of pharmaceutical products.
Understanding the overall manufacturing chain as an interconnected system calls for fundamentally different ways of thinking and working. Along with new technical competencies (how to use the tools and technologies), the holistic approach also includes a broad range of people factors, such as aspects of change management (e.g., critical mass awareness and acceptance of new approaches), knowledge and skills development in the current and future workforce, and overcoming any (usually unintended) silo behaviors between functions and supply chain connections, including geographical challenges of global manufacturing organizations.
To succeed with a digital approach, the organization must implement a substantial cultural change that supports the move from old habits (using modeling to explain experimental results) to new (using models to ensure practical outcomes and identify experiments to verify outcomes). To succeed, the organization’s approach to recruitment and training must change, so it can develop scientists and engineers capable as experimentalists and model-ers, rather than perpetuating two separate communities.
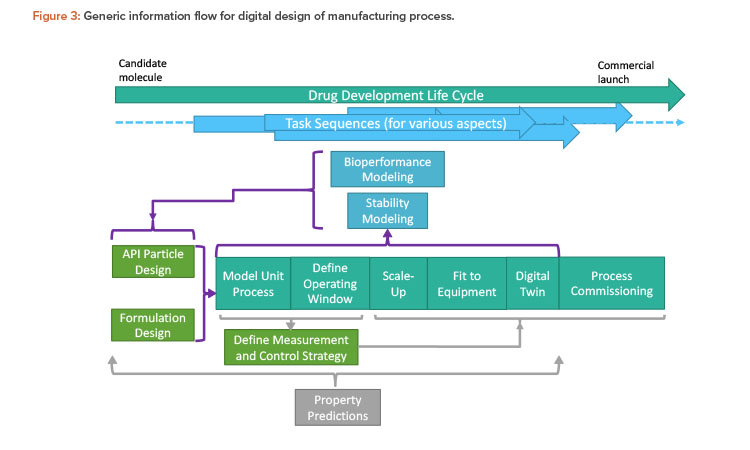
Successful information flow must also highlight key risk-assessment hot spots, business and route decision points, and the information requirements for facilitating them. Digital design enables much earlier integration of measurement and control strategies in equipment design, with the aim of achieving more robust, controllable manufacturing processes. In turn, early understanding of measurement and control enables the development of a manufacturing process “digital twin,” where mechanistic system models are used to enable dynamic simulation of the whole process, not merely individual steps. Such an approach embodies the principles behind QbD, making it part of the “way things get done,” rather than an additional consideration to discuss during filing.
ADDoPT Information Flow Architecture
In the ADDoPT approach, understanding of the entire pharmaceutical information flow process begins when software developers and users answer the following questions together:
- Which specific tasks, operations, and decisions are addressed by digital modeling?
- What is the level of model fidelity? What are the model’s assumptions and limitations? Are those assumptions and limitations appropriate to the application scope?
- What data are required to run a model? What are the outputs? (These matters are key to managing interfaces between different modeling components.)
- Where does the modeling need to be applied? Who will be applying it, and what skills do they need?
Answers to these questions provide the conceptual framework within which developers and users can co-create solutions that anticipate the upstream and downstream impacts of design decisions and best fit the needs of the industry.
Britest’s existing whole-process understanding tools were used to help developers and users address the questions in a structured way and ensure mutual communication and comprehension of the issues and potential solutions. In developing the information flow, some simplifying constraints have been imposed to maintain a manageable scope. The starting point is a small molecule API with a synthesis route defined elsewhere that will be delivered as a solid oral dose. The requirements defined in Figure 2 are addressed by the overall information flow structure for digital design (Figure 3).
The overall drug development life cycle runs from selection of the candidate molecule to commercial launch, including the project timeline and stage gates (e.g., first through third clinical trials). The task sequences are the individual tasks required to obtain the deliverables necessary for the project stage gates. These sequences are usually carried out by separate groups within the organization. The key task sequences are illustrated, with inter-connecting information flows indicated by brackets and arrows. For example, in Figure 3, the defining steps are API particle design and formulation design, which together encompass product design. Here, the requirements for efficacy, stability, and patient safety should come together with consideration of manufacturability to deliver a whole-process design running through the information flow. This, in turn, feeds back to the bioperformance and stability areas, enabling product design to be reassessed and optimized in a “virtuous cycle” enabled by extensive use of modeling at all points.
The strategy for measurement and control is informed by and supports the process design. Modeling underpins everything to provide physical property estimates for all models employed in all preceding task sequences.
The pharmaceutical industry needs to integrate process development with considerations of patient safety, efficacy, and manufacturability.
The information flow can be documented as an interactive flowchart with hyperlinks so it’s easy to jump from task to task. An e-learning solution (Articulate Storyline) facilitates layering information from a high-level overview to increased depth and detail, and provides web-enabled output, which is ideal for dissemination. To allow greater flexibility in exploring the link between available models and tasks, a visual relational database interface (SharpCloud) has been interwoven with the e-learning solution.
Implicit within the information flow requirements is the desire to make the information flow more practical for implementation by annotating clear explanations and descriptions of alternative models that could be used to achieve tasks identified, and to signpost training, support, and service providers who can help industrialists put them in practice. In an in-house implementation, this documentation could be expanded to include contact details for subject matter experts. Thus, information flow development has become confluent with the development of an ADDoPT online Digital Design Guide, within which it will ultimately be embedded as a primary piece of the publicly available project.
Dissemination and Exploitation of the Addopt Information Flow
The ADDoPT information flow has been developed to be general in application for the global pharmaceutical sector. In this form, it is an aid for SMEs to position services supporting digital design and manufacture. For pharmaceutical companies, and the contract research and manufacturing organizations in their supply chains, it will provide a resource for introducing researchers to the value and opportunities of digital design and modeling. These outputs will be generally accessible through publications and an open-access website.1
For implementation within individual organizations, further work will be required to tailor the information flow to the organization’s needs. Customization is required to adapt language and tasks to different business processes, cultures, and priorities, and will also consider the different models used, including special models developed in-house.
It is believed that an independently facilitated process will be the most efficient way for a business to develop a bespoke information flow to realize the advantages of digital design and manufacture while incurring minimal disruption during changeover. Within ADDoPT, Britest has already used the methodology to deliver bespoke, visually accessible frameworks for the application of digital model tools to two of the major pharmaceutical partners in the ADDoPT project.
Conclusion
The ADDoPT consortium has developed information flow for digital design and manufacture of pharmaceutical products. This innovation will help the global pharmaceutical industry implement the Made Smarter Review’s recommendations regarding technology adoption, innovation, and leadership, which have been identified as essential to realize the potential of the Industry 4.0 revolution.
The generic information flow developed is a template that can be customized to meet individual organizational needs. This is best done via an independently facilitated workshop-based process identifying existing modeling capability and gaps. Similar information flows for digital design and manufacture will be applicable outside the pharmaceutical industry, and the pharmaceutical information flow can act as a template of this digital-enabling approach for other areas of manufacturing.
Acknowledgments
The ADDoPT collaboration was initiated by the Medicines Manufacturing Industry Partnership (MMIP) and partially funded under the Advanced Manufacturing Supply Chain Initiative, a UK Department of Business, Energy and Industrial Strategy initiative delivered by Finance Birmingham and Birmingham City Council. The authors are grateful to colleagues from across the ADDoPT consortium for their input to workshop sessions and various helpful discussions in the development of the approach described herein.